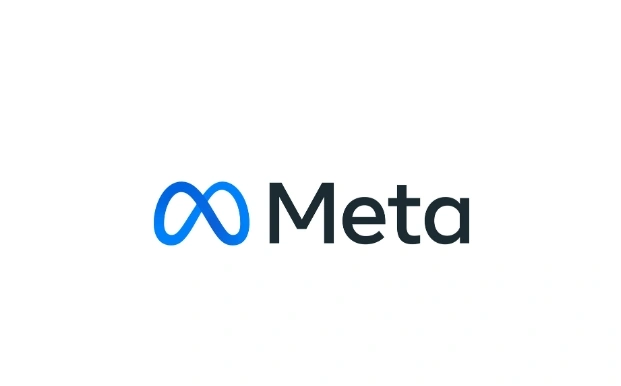
The FAIR team under Meta AI, in collaboration with Georgia Tech, jointly developed the CATransformers framework, taking carbon emissions into account as a core design consideration. By jointly optimizing model architecture and hardware performance, total carbon emissions are significantly reduced, marking an important step towards sustainable AI development.
The widespread adoption of machine learning technologies has driven innovations ranging from recommendation systems to autonomous driving, but their environmental cost cannot be underestimated. These systems require powerful computing resources and often rely on customized hardware accelerators to run. The large energy consumption during the training and inference stages directly translates into increased operational carbon emissions.
In addition, the entire lifecycle of hardware, from manufacturing to disposal, also generates "embedded carbon," further increasing the environmental burden. As the adoption of AI technologies accelerates in industries around the world, it is urgent to address the dual sources of emissions: operational and embedded carbon.
Current approaches to reduce emissions are primarily focused on improving operational efficiency, such as optimizing energy consumption for training and inference, and improving hardware utilization. However, these methods often ignore carbon emissions during the hardware design and manufacturing stages and fail to integrate the mutual impact of model design and hardware efficiency.
The CATransformers framework, jointly launched by Meta's FAIR team and Georgia Tech, incorporates carbon emissions into its core design considerations. The framework jointly evaluates model architectures and hardware accelerator performance through a multi-objective Bayesian optimization engine to balance latency, energy consumption, accuracy, and total carbon emissions.
Specifically for edge inference devices, CATransformers reduces large CLIP models to generate variants and combines them with hardware estimators to analyze carbon emissions and performance. As a result, CarbonCLIP-S has the same accuracy as TinyCLIP-39M, while reducing carbon emissions by 17% and reducing latency to less than 15 ms. CarbonCLIP-XS achieves 8% accuracy improvement, 3% carbon footprint reduction, and less than 10ms latency over TinyCLIP-8M.
Research shows that designs that optimize latency alone can result in up to 2.4x higher carbon emissions, while a combined carbon footprint optimization and latency strategy can reduce total emissions by 19-20% while minimizing latency penalties.
By embedding environmental metrics, CATransformers lays the foundation for the design of sustainable machine learning systems. This shows that combining hardware capabilities with carbon impact considerations from the start in AI development can provide benefits in both performance and sustainability. As AI continues to expand, this framework provides the industry with a practical path to reduce emissions.